Data Integration
What is difference between data integration and data fusion?
There is a lot of confusion between data integration and data fusion, but there is a big difference between the two. Data integration is the process of combining data from different sources into a single database or data mart. This can be done by matching fields in the data sources or by importing the data into a common format. Data fusion, on the other hand, is the process of combining information from multiple sources to create a more complete picture. This can be done by identifying common elements among the data sources or by synthesizing the data into a new, composite source.
Data fusion typically produces a more accurate picture than data integration because it takes into account all of the available information. Data integration can only combine data that is identical or compatible, while data fusion can combine data that is dissimilar. Data fusion is also better at detecting patterns and identifying relationships among the data sources. As a result, data fusion can be used to create more accurate models and predictions.
Data integration is typically used for operational purposes, such as creating a single source of truth for reporting or analytics. Data fusion is more often used for research and discovery, where a more complete understanding of the data is required. However, the two techniques can be complementary, and data fusion can be used to improve the results of data integration. In the end, it is up to the individual organization to decide which approach works best for them.
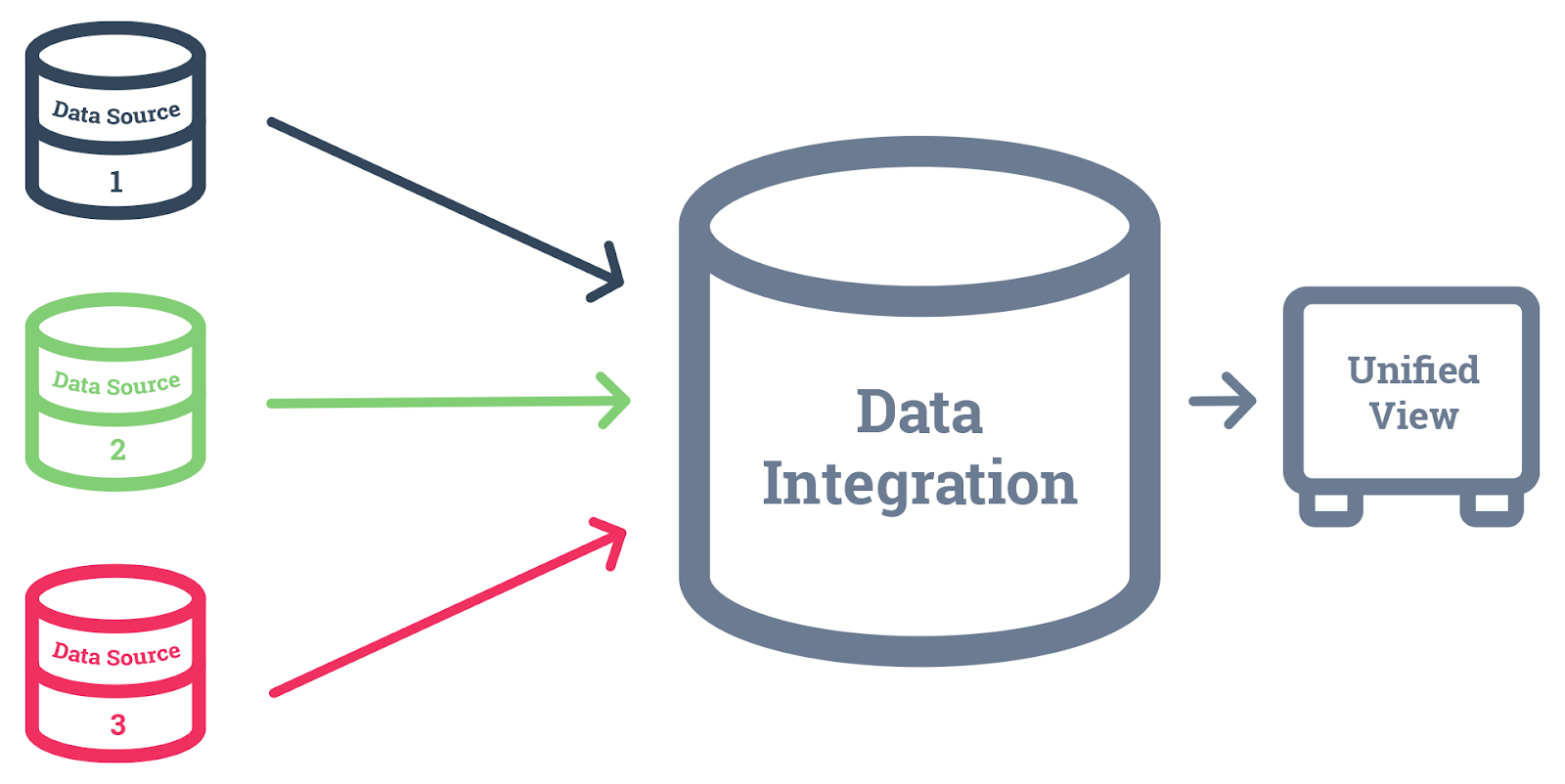
What are the differences between data integration and big data?
Data integration is the process of combining data from disparate sources into a cohesive, unified whole. Big data is a term for data sets that are so large and complex that traditional data-processing methods are unable to handle them.
The main difference between data integration and big data is that data integration is a process of combining data from disparate sources into a cohesive, unified whole, while big data is a term for data sets that are so large and complex that traditional data-processing methods are unable to handle them.
Data integration can be used to combine data from multiple sources into a single repository, such as a data warehouse. This can make it easier to analyze the data and look for patterns. Big data, on the other hand, generally refers to datasets that are too large or complex to be processed using traditional methods.
There is some overlap between the two concepts, as big data sets often need to be integrated from multiple sources. However, not all big data sets require integration, and not all data integrations will be large enough to be considered big data.
The main difference between data integration and big data is that data integration is the process of combining data from disparate sources into a cohesive, unified whole, while big data is a term for data sets that are so large and complex that traditional data-processing methods are unable to handle them. Data integration can be used to combine data from multiple sources into a single repository, such as a data warehouse. This can make it easier to analyze the data and look for patterns. Big data, on the other hand, generally refers to datasets that are too large or complex to be processed using traditional methods. There is some overlap between the two concepts, as big data sets often need to be integrated from multiple sources. However, not all big data sets require integration, and not all data integrations will be large enough to be considered big data.
In general, data integration is concerned with combining data from different sources into a single repository. The goal is to make it easier to analyze the data and look for patterns. Big data, on the other hand, is generally defined as datasets that are too large or complex to be processed using traditional methods. There is some overlap between the two concepts, as big data sets often need to be integrated from multiple sources. However, not all big data sets require integration, and not all data integrations will be large enough to be considered big data.
What is data integration software?
Data integration software is a type of software that helps to combine data from different sources into one central location. This can be helpful for businesses that need to consolidate data from multiple sources in order to make better decisions. Data integration software can also help to improve the efficiency of Manchester business processes by consolidating data in one place. There are many different types of data integration software available, and choosing the right one can be a challenge. This article will provide an overview of some of the most popular data integration software products on the market.
Some of the most popular data integration software products include:
– IBM InfoSphere DataStage
– Oracle Data Integrator
– SAP Data Services
– Informatica PowerCenter
– Talend Open Studio for Data Integration
Each of these products has its own unique features and benefits, so it is important to select the one that best meets the needs of your Manchester organization. In general, data integration software products can be categorized into two main types: ETL tools and ELT tools. ETL tools are designed to extract data from source systems, transform it, and load it into a target system. ELT tools are designed to do the same thing, but they also include a database or data warehouse in which the data can be stored. This can be helpful for businesses that need to keep data for long-term storage or analysis.
Choosing the right data integration software can be a daunting task, but it is important to select a product that meets the specific needs of your organization. By doing so, you can improve the efficiency and effectiveness of your business operations. Contact Manchester Apps and let us help you choose the right data integration software.